Why do some organizations bounce back from crises stronger, while others strugg....
Navigating ISO Standards and AI Governance for a Secure Future
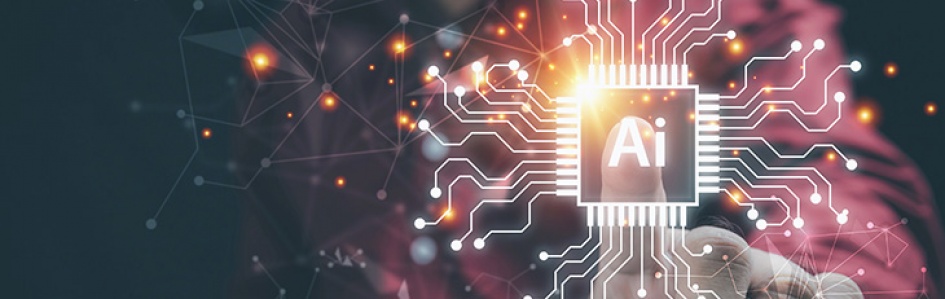
In times when artificial intelligence (AI) is reshaping the landscape of technology and business, the importance of robust governance frameworks cannot be overstated. Adhering to ISO standards becomes crucial for organizations aiming to harness AI's potential responsibly. This ensures that their AI systems are not only efficient and effective but also safe and trustworthy.
This article delves into the intricate interplay between ISO standards and AI governance, clarifying how they form the foundation of a secure and sustainable future.
Understanding ISO Standards
ISO standards, developed and published by ISO are globally recognized frameworks that establish guidelines, specifications, and requirements for various processes, products, and services across different industries. These standards aim to ensure quality, efficiency, safety, and interoperability in both products and processes. They serve as a benchmark for organizations to adhere to, facilitating consistency, reliability, and compatibility in their operations.
The development process of ISO standards for AI technologies is a thorough and collaborative effort involving experts, stakeholders, and industry representatives from around the world. Given the rapid advancements and complex nature of artificial intelligence, developing ISO standards for AI requires thorough research, analysis of best practices, and consideration of ethical, legal, and societal implications.
This process involves several stages:
- Initial proposal
- Drafting
- Committee review
- Public consultation
- Finalization
These stages ensure that the standards reflect the latest technological advancements and address emerging challenges in the field of AI.
Key ISO standards relevant to AI encompass various aspects of information security, data management, and governance. Among the prominent ISO standards relevant to AI are ISO/IEC 42001 and ISO/IEC 27001.
ISO/IEC 42001 Artificial Intelligence Management System
ISO/IEC 42001:2023 is a significant milestone in the field of artificial intelligence, marking the introduction of a standardized framework for Artificial Intelligence Management Systems (AIMS).
This international standard provides comprehensive guidelines for organizations to establish, implement, maintain, and continually improve their AIMS. It is designed to address the unique challenges posed by AI technologies, including ethical considerations, transparency, and the need for continuous learning and adaptation.
The standard is applicable to organizations across various industries and sectors and it helps in managing risks and opportunities associated with AI, ensuring that innovation is balanced with governance and ethical responsibility. It also aligns with global initiatives and regulations that advocate for ethical AI development, emphasizing fairness, accountability, and security.
The Role of ISO/IEC 27001 in AI Management
ISO/IEC 27001:2022 is a globally recognized standard that outlines the requirements for establishing, implementing, maintaining, and continually improving an Information Security Management System (ISMS).
This framework is designed to help organizations secure their information assets, such as financial information, intellectual properties, employee details, or information entrusted by third parties.
AI systems process vast amounts of data and have the potential to significantly impact the security posture of an organization. By adhering to the principles of ISO/IEC 27001, AI technologies can be governed to ensure they maintain confidentiality, integrity, and availability of data—often referred to as the CIA triad.
In the context of AI, ISO/IEC 27001 can provide a structured approach to managing risks associated with AI technologies. This includes ensuring that AI systems are designed and operate in a manner that respects privacy rights and is free from biases that could lead to unfair treatment or discrimination.
The standard encourages a holistic approach to security, considering not just technical measures, but also organizational processes and human factors. For instance, it can guide the development of policies for AI ethics, data protection impact assessments, and the monitoring of AI systems to detect and respond to security incidents promptly.
As organizations embrace the principles of ISO/IEC 27001 for comprehensive security, the symbiotic relationship between artificial intelligence (AI) and the standard becomes increasingly apparent.
AI Governance
AI governance ensures ethical, safe, and responsible development and deployment of artificial intelligence technologies. It encompasses a set of rules, standards, and processes that guide AI research and applications, aiming to protect human rights and promote fairness, accountability, and transparency.
The significance of AI governance lies in its ability to address the inherent risks associated with AI, such as bias, privacy violation, and misuse, which can lead to social and ethical harm. By implementing robust AI governance, organizations and governments can foster innovation while maintaining public trust and compliance with regulatory requirements. Moreover, AI governance is essential for aligning AI behaviors with societal values and ethical standards, thus, safeguarding against potential adverse impacts on individuals and communities.
Current Challenges in AI Governance
AI governance is grappling with a multitude of challenges as it strives to keep pace with the rapid advancement of technology. A primary concern is the rapidity of AI developments, which often surpasses regulatory frameworks' adaptability, leaving AI operating with inadequate oversight. This lack of regulation raises serious issues regarding accountability and the ethical use of AI.
Another challenge is the potential for AI to intensify issues like misinformation, deepfakes, surveillance, and other forms of online harm. These risks necessitate a governance approach that not only harnesses the benefits of AI but also establishes ways to mitigate its risks.
Institutional inertia is another difficulty, as government bodies may prioritize more immediate political concerns over AI governance, leading to a loss of urgency and focus on the issue. Furthermore, the disparity between the pace of AI breakthroughs and the advancement in governance is widening, with political dynamics and the fragmented approach to evaluating foundational AI models contributing to the governance gap.
The proposition of an Intergovernmental Panel on Climate Change (IPCC)-like body for AI represents a positive step toward a unified global scientific understanding of AI and its societal and political implications.
Security Threats Specific to AI Systems
While transformative in their capabilities, AI systems are not immune to security threats. Here are some AI-specific security threats:
Data Poisoning
Data poisoning is a critical security concern in the field of artificial intelligence (AI) and machine learning (ML), where malicious actors intentionally manipulate training data to compromise the integrity of AI models. For instance, attackers can insert malware into the system, as was the case with 100 poisoned models found on the Hugging Face AI platform.
This can be achieved through various methods such as injecting false data, modifying existing data, or deleting portions of the dataset. The consequences of such attacks can be severe, leading to biased results, degraded model performance, and the introduction of vulnerabilities that could be exploited.
To mitigate data poisoning, it is essential to implement robust data validation and sanitization processes. Additionally, employing anomaly detection algorithms can help monitor the data in real-time, flagging potential issues for review.
Model Stealing
Model stealing refers to the unauthorized replication of a machine learning model by exploiting its input-output relationship. This is often done by querying the target model with various inputs and using the outputs to reconstruct a similar model. The implications of such attacks are considerable, as they can undermine the investment and intellectual property associated with the development of the original model.
For example, model stealing in online games involves unauthorized replication of game assets and exploitation of game systems for financial gain, as seen in Fortnite where criminals exploit stolen bank card details to purchase and resell in-game currency.
To combat model stealing, one could implement several strategies:
- Limit the number of queries to prevent data collection for model replication.
- Add random noise to outputs to confound attackers.
- Use watermarking techniques to embed unique patterns in predictions for ownership tracing.
- Implement legal agreements and robust cybersecurity measures as deterrents and additional protection.
Model Inversion Attacks
Model Inversion Attacks (MIAs) are a type of cybersecurity threat targeting machine learning models. The purpose of these attacks is to reverse engineer the outputs of a model in order to infer sensitive information about its training data. This can be particularly concerning when the model has been trained on personal data, such as images in a facial recognition system. The risk associated with MIAs is significant, as they can potentially compromise the confidentiality of sensitive data and violate privacy regulations.
A real-life example of an MIA was demonstrated by Fredrikson et al. in 2015, where researchers were able to reconstruct facial images from a machine learning model that was trained to predict demographic data. This attack highlighted the vulnerability of machine learning models to reveal sensitive information, even from non-sensitive predictions.
To mitigate Model Inversion Attacks, it is crucial to implement robust security measures:
- Utilize differential privacy techniques to add noise to model outputs, hindering attackers' ability to reconstruct input data accurately.
- Regularly retrain models with updated data to prevent exploitation of outdated information.
- Employ encryption for data at rest and in transit to maintain data confidentiality.
- Implement access controls and thorough auditing to detect and prevent unauthorized attempts to probe machine learning models.
In conclusion, the convergence of ISO standards and AI governance heralds a new era of responsible innovation and ethical deployment practices. By navigating these frameworks synergistically, organizations can forge a path toward a secure and sustainable future.
As we continue to navigate the evolving landscape of technology and ethics, it is imperative to uphold the principles of accountability, transparency, and ethical responsibility. This ensures AI contributes positively to our interconnected world.
For a deeper insight from industry experts, explore our previous webinar: Securing the Future: ISO/IEC 27001, ISO/IEC 42001, and AI Governance.
You can also get certified on:
- ISO/IEC 27001 Lead Implementer
- ISO/IEC 27001 Lead Auditor
- ISO/IEC 27001 Transition
- ISO/IEC 42001 Lead Implementer
- ISO/IEC 42001 Lead Auditor
About the Author
Vlerë Hyseni is the Digital Content Specialist at PECB. She is in charge of doing research, creating, and developing digital content for a variety of industries. If you have any questions, please do not hesitate to contact her at: content@pecb.com.